Goal setting should be an exercise in prediction, but in reality it becomes more of a negotiation between marketing and finance. Marketers want a lower target and more budget, so they âsandbagâ the numbers, meaning they give themselves some wiggle room versus what they actually predict will happen. Finance wants a higher target and less budget, so they interrogate the budget, diligently looking for waste vs industry benchmarks of whatâs ânormalâ to spend.Â
The stakes couldnât be higher: team bonuses are tied to hitting goals, and if the CMO misses the target by too much, they could be looking for another job. If finance gets it wrong and over-allocates budget to marketing that could have had a higher return elsewhere, results for the whole business will suffer. This great game usually takes place in a Microsoft Excel spreadsheet. Assumptions are made, numbers are extrapolated, and the result is a model of how the business makes money.Â
Marketing is just one of the factors this spreadsheet has to account for, and changing one factor affects every other. For example an increase in marketing spend might require increased headcount for customer service, advanceds purchasing more raw materials, or investing in a bigger robot for the factory. If marketing misses their number, thereâs a cascading effect as the business doesnât have the demand it was preparing for. If youâre wrong by a lot, the business might even be at risk of bankruptcy.
The problem with financial models
The often quoted George Box said âAll models are wrong, some are usefulâ. Models by their very nature are simplifications of reality. We know that the numbers in the spreadsheet donât account for everything. However these financial models are useful as a management tool: they help us make decisions under uncertainty. But ironically thatâs the one thing most models get wrong â they donât factor in uncertainty!Â
Take a simple model of marketing efficiency. Historically your CPA (Cost Per Acquisition) for Google Ads has been $130 on average. So in your model you would take your budget, and divide by the CPA, get the contribution to new customers you can expect from that channel. For example if you spend $100,000 you would get 100,000 / 130 = 769 new customers. That type of simplistic marketing funnel calculation features in most every budget spreadsheet.
We said our CPA has been $130 on average, but that precise figure ignores uncertainty. It could have varied wildly between $90 and $170, or it could have been relatively stable between $120 and $140 â our model has no idea. Some channels will be more stable than others. Some campaigns might have a $100 CPA, and others might be closer to $150. This matters because if we go and push more budget into the channel, and that CPA assumption wasnât very robust, we could very well end up with far fewer customers than we expected, missing our target.
How to be less wrong
The correct approach is to factor in uncertainty using something called a Monte-Carlo simulation. This is a technique invented by Stan Ulam, one of the fathers of the Manhattan Project that produced the Atomic Bomb. He was trying to estimate his chances of winning games of Solitaire, and realized he couldnât derive the formulas for predicting the odds, it was too complex. Instead if you âbrute forceâ an approach by making a computer play thousands of games, simulating all possible outcomes, you can simply count the successful games and divide by unsuccessful outcomes to get your resulting probability of winning.
â
The way it would work in our model example is as follows. Instead of your $130 CPA, you input a range, say $100 to $200. Rather than simply dividing your budget by $130 to get a single answer for the number of impressions served, you divide by a range, or probability distribution. Now you know that your $100,000 could buy you anywhere from 1,000 to 2,000 new customers. You donât get one answer, you get a distribution of potential outcomes, from which you can count the likelihood of each potential scenario you want to predict. Now you can go to finance and rather than promising them youâll hit some precise number, you can give them the likelihood of hitting it with the resource given.
This is a subtle change but it makes a world of difference. Rather than a tug-of-war negotiation, goal setting becomes a more honest collaboration. When you can say confidently âwith $100,000 weâre 90% likely to hit the targetâ, that budget request is easier to approve. When you warn that âcutting the budget to $80,000 brings our odds of hitting the target down to 60%â, you can have a more informed conversation with leadership about whatâs realistic. When youâre open about uncertainty, you can spot places in the model where uncertainty is the highest. These are usually hidden assumptions that differ amongst teams, maybe even between individual members of the team.Â
When the Google Ads specialist says CPA will be between $120 and $140, thatâs a reflection in their confidence. If the Facebook Ads specialist gives a range of $50 to $200, that shows everyone how shaky that assumption is. We can avoid putting all our hope in Facebook to hit our numbers, and justify investment into further testing to reduce that uncertainty. Itâs possible to do this in Excel but itâs clunky, and a lot of extra work, so most donât do it. Thankfully there are next-generation tools like Causal that incorporate this functionality right out of the box. Weâll now walk through a real world scenario where this approach comes in handy.
Marketing Mix Modeling in Causal
One area this approach becomes essential is in Marketing Mix Modeling: a probabilistic approach to modeling business outcomes from marketing. This is a technique from the 1960s that rose to prominence in public companies due to Sarbannes-Oxleyâs requirement to justify large expenditures like advertising budgets. By matching spikes and dips in sales to activities and events in marketing with regression analysis, you can build a predictive model of what levers drive sales for the business.
It is gaining popularity even with smaller startups today, because it doesnât rely on user-level data, making it âprivacy friendlyâ and future proof to data governance issues like GPDRâs privacy legislation, the rise of Ad blockers, and Appleâs Tracking prevention measures released in iOS14. However, being a probabilistic method, there can be a great deal of uncertainty to deal with, that marketers arenât used to modeling. Thatâs where a tool like Causal that lets you bake in uncertainty to the model can really help.Â
Below is an example of a traditional marketing mix model, which you could build in Excel or Google Sheets using the LINEST function, a Linear Regression estimator.
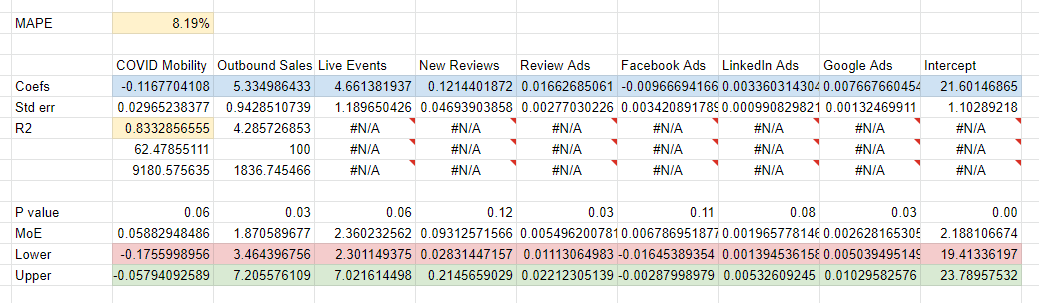
In this model the Coefs, the row in blue, refer to the coefficients. This model is saying that to find the contribution of your Google Ads, you need to multiply 0.007667 by the amount you spent, to get the number of customers it drove. So if we spent $100,000 we drove 766 customers, which in this case as it was a B2B client, refers to the number of Leads. We can work out the CPA by dividing 1 by the coefficient, so 1 / 0.007667 = $130. The other part of the model we want to focus on are the Lower and Upper bounds, represented in the red and green lines. This is where we get the uncertainty from. So for the upper bound we have 1 / 0.01029 = $97 CPA, and at the lower bound we have 1 / 0.00503 = $198 CPA. This is a pretty big range, so if we only estimated the impact of Google Ads using the $130 CPA figure, we could be wrong by a lot.

Above is how we would represent that uncertainty in Causal. Weâve added the range of upper to lower bounds into the model, rather than a fixed static number, for each of the model coefficients. Causal displays the average using a triangle distribution to calculate it, but when calculating downstream outputs of the model it does so by multiplying the full range. We work out the contribution for each channel by taking the input (how much we plan to spend) and multiplying it by this β Google Ads variable. When you hover over the cell you see the range of potential outcomes made by the prediction.
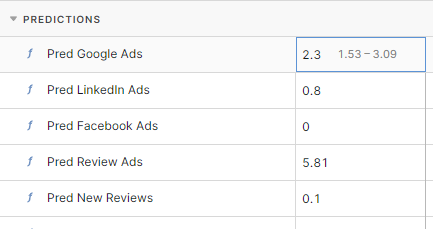
Finally the whole model comes together at the end in the outcome: the prediction of how many Leads weâll get given our planned marketing spend. This is what weâve been working towards: a probability breakdown of what number of Leads weâre likely to achieve based on the uncertainty inherent in our model. By default in Causal you get the 90% probability range, so you can tell with good certainty what outcome is most certain.
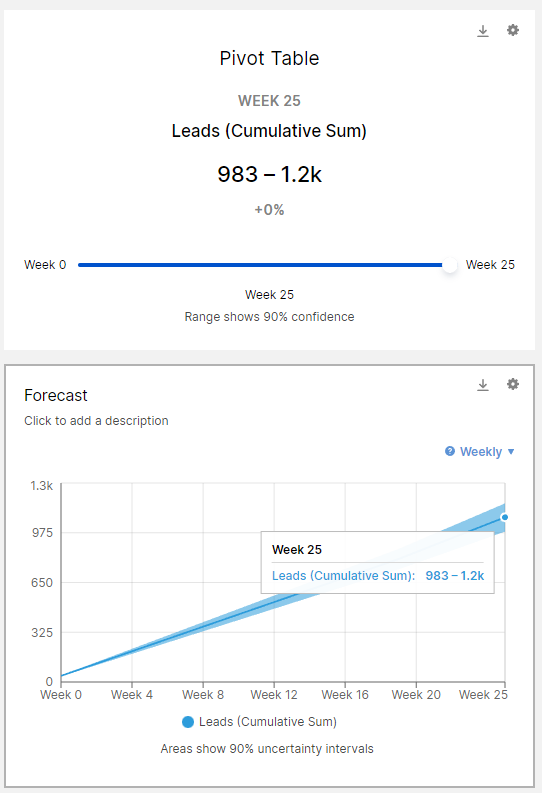
As you forecast out further in time the uncertainties pile up, so the potential outcome range gets wider. With the existing input of marketing spend this model is telling me not a specific number, but a range: weâre 90% certain weâll get at least 983 Leads, and maybe as much as 1.2k. If thatâs not good enough, we can make adjustments to our budget inputs and see the result. This way of thinking probabilistically doesnât come naturally: we like static numbers and try and hide from uncertainty. However if youâre up front with it from the very beginning, you can make better, more honest decisions, and be more confident that youâre setting realistic expectations around what goals are achievable, and what are likely to be missed.
Of course this is just a simple marketing mix model, for demonstration purposes. If you were doing this for real you might want to try a Bayesian approach like what we use at Recast. It actually uses Monte Carlo simulation under the hood to help build the model, though at a far larger scale than the range calculations made by Causal. Rather than the upper and lower bounds we used as an analogy for uncertainty, with a Bayesian model you get the underlying probability range of each variable in your model, which can be plugged directly into Causal.
Weâve provided the Marketing Mix Model in Google Sheets and the Causal Model Template below.. If you want to try out this Causal model, click through on the link below to clone the template, and create an account if you donât have one.Â